Abstract
A major challenge in human genetics is to devise a systematic strategy to integrate disease-associated variants with diverse genomic and biological data sets to provide insight into disease pathogenesis and guide drug discovery for complex traits such as rheumatoid arthritis (RA)1. Here we performed a genome-wide association study meta-analysis in a total of >100,000 subjects of European and Asian ancestries (29,880 RA cases and 73,758 controls), by evaluating ∼10 million single-nucleotide polymorphisms. We discovered 42 novel RA risk loci at a genome-wide level of significance, bringing the total to 101 (refs 2, 3, 4). We devised an in silico pipeline using established bioinformatics methods based on functional annotation5, cis-acting expression quantitative trait loci6 and pathway analyses7,8,9—as well as novel methods based on genetic overlap with human primary immunodeficiency, haematological cancer somatic mutations and knockout mouse phenotypes—to identify 98 biological candidate genes at these 101 risk loci. We demonstrate that these genes are the targets of approved therapies for RA, and further suggest that drugs approved for other indications may be repurposed for the treatment of RA. Together, this comprehensive genetic study sheds light on fundamental genes, pathways and cell types that contribute to RA pathogenesis, and provides empirical evidence that the genetics of RA can provide important information for drug discovery.
This is a preview of subscription content, access via your institution
Access options
Subscribe to this journal
Receive 51 print issues and online access
$199.00 per year
only $3.90 per issue
Buy this article
- Purchase on SpringerLink
- Instant access to full article PDF
Prices may be subject to local taxes which are calculated during checkout



Similar content being viewed by others
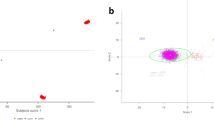
References
Plenge, R. M., Scolnick, E. M. & Altshuler, D. Validating therapeutic targets through human genetics. Nature Rev. Drug Discov. 12, 581–594 (2013)
Stahl, E. A. et al. Genome-wide association study meta-analysis identifies seven new rheumatoid arthritis risk loci. Nature Genet. 42, 508–514 (2010)
Okada, Y. et al. Meta-analysis identifies nine new loci associated with rheumatoid arthritis in the Japanese population. Nature Genet. 44, 511–516 (2012)
Eyre, S. et al. High-density genetic mapping identifies new susceptibility loci for rheumatoid arthritis. Nature Genet. 44, 1336–1340 (2012)
Ferreira, R. C. et al. Functional IL6R 358Ala allele impairs classical IL-6 receptor signaling and influences risk of diverse inflammatory diseases. PLoS Genet. 9, e1003444 (2013)
Westra, H. J. et al. Systematic identification of trans eQTLs as putative drivers of known disease associations. Nature Genet. 45, 1238–1243 (2013)
Raychaudhuri, S. et al. Identifying relationships among genomic disease regions: predicting genes at pathogenic SNP associations and rare deletions. PLoS Genet. 5, e1000534 (2009)
Rossin, E. J. et al. Proteins encoded in genomic regions associated with immune-mediated disease physically interact and suggest underlying biology. PLoS Genet. 7, e1001273 (2011)
Segrè, A. V., Groop, L., Mootha, V. K., Daly, M. J. & Altshuler, D. Common inherited variation in mitochondrial genes is not enriched for associations with type 2 diabetes or related glycemic traits. PLoS Genet. 6, e1001058 (2010)
Stahl, E. A. et al. Bayesian inference analyses of the polygenic architecture of rheumatoid arthritis. Nature Genet. 44, 483–489 (2012)
1000 Genomes Project Consortium et al. An integrated map of genetic variation from 1,092 human genomes. Nature 491, 56–65 (2012)
Raychaudhuri, S. et al. Five amino acids in three HLA proteins explain most of the association between MHC and seropositive rheumatoid arthritis. Nature Genet. 44, 291–296 (2012)
Trynka, G. et al. Chromatin marks identify critical cell types for fine mapping complex trait variants. Nature Genet. 45, 124–130 (2013)
Parvaneh, N., Casanova, J. L., Notarangelo, L. D. & Conley, M. E. Primary immunodeficiencies: a rapidly evolving story. J. Allergy Clin. Immunol. 131, 314–323 (2013)
Forbes, S. A. et al. COSMIC: mining complete cancer genomes in the Catalogue of Somatic Mutations in Cancer. Nucleic Acids Res. 39, D945–D950 (2011)
Eppig, J. T., Blake, J. A., Bult, C. J., Kadin, J. A. & Richardson, J. E. The Mouse Genome Database (MGD): comprehensive resource for genetics and genomics of the laboratory mouse. Nucleic Acids Res. 40, D881–D886 (2012)
Knox, C. et al. DrugBank 3.0: a comprehensive resource for ‘omics’ research on drugs. Nucleic Acids Res. 39, D1035–D1041 (2011)
Zhu, F. et al. Therapeutic target database update 2012: a resource for facilitating target-oriented drug discovery. Nucleic Acids Res. 40, D1128–D1136 (2012)
Smolen, J. S. et al. Consensus statement on blocking the effects of interleukin-6 and in particular by interleukin-6 receptor inhibition in rheumatoid arthritis and other inflammatory conditions. Ann. Rheum. Dis. 72, 482–492 (2013)
Nishimoto, N. et al. Study of active controlled tocilizumab monotherapy for rheumatoid arthritis patients with an inadequate response to methotrexate (SATORI): significant reduction in disease activity and serum vascular endothelial growth factor by IL-6 receptor inhibition therapy. Mod. Rheumatol. 19, 12–19 (2009)
McInnes, I. B. & Schett, G. The pathogenesis of rheumatoid arthritis. N. Engl. J. Med. 365, 2205–2219 (2011)
Sekine, C. et al. Successful treatment of animal models of rheumatoid arthritis with small-molecule cyclin-dependent kinase inhibitors. J. Immunol. 180, 1954–1961 (2008)
Sanseau, P. et al. Use of genome-wide association studies for drug repositioning. Nature Biotechnol. 30, 317–320 (2012)
Arnett, F. C. et al. The American Rheumatism Association 1987 revised criteria for the classification of rheumatoid arthritis. Arthritis Rheum. 31, 315–324 (1988)
Okada, Y. et al. Meta-analysis identifies multiple loci associated with kidney function-related traits in east Asian populations. Nature Genet. 44, 904–909 (2012)
Hindorff, L. A. et al. Potential etiologic and functional implications of genome-wide association loci for human diseases and traits. Proc. Natl Acad. Sci. USA 106, 9362–9367 (2009)
Lage, K. et al. A human phenome-interactome network of protein complexes implicated in genetic disorders. Nature Biotechnol. 25, 309–316 (2007)
Ueda, H. et al. Association of the T-cell regulatory gene CTLA4 with susceptibility to autoimmune disease. Nature 423, 506–511 (2003)
Elliott, P. et al. Genetic loci associated with C-reactive protein levels and risk of coronary heart disease. J. Am. Med. Assoc. 302, 37–48 (2009)
Cortes, A. et al. Identification of multiple risk variants for ankylosing spondylitis through high-density genotyping of immune-related loci. Nature Genet. 45, 730–738 (2013)
Acknowledgements
R.M.P. is supported by National Institutes of Health (NIH) grants R01-AR057108, R01-AR056768, U01-GM092691 and R01-AR059648, and holds a Career Award for Medical Scientists from the Burroughs Wellcome Fund. Y.O. is supported by a grant from the Japan Society of the Promotion of Science. D.W. is supported by a grant from the Australian National Health and Medical Research Council (1036541). G.T. is supported by the Rubicon grant from the Netherlands Organization for Scientific Research. A.Z. is supported by a grant from the Dutch Reumafonds (11-1-101) and from the Rosalind Franklin Fellowship, University of Groningen. S.-C.B., S.-Y.B. and H.-S.L. are supported by the Korea Healthcare technology R&D project, Ministry for Health and Welfare (A121983). J.M., M.A.G.-G. and L.R.-R. are funded by the RETICS program, RIER, RD12/0009 from the Instituto de Salud Carlos III, Health Ministry. S.R.-D. and L.Ä.’s work is supported by the Medical Biobank of Northern Sweden. H.K.C. is supported by NIH (NIAMS) grants R01-AR056291, R01-AR065944, R01-AR056768, P60 AR047785 and R21 AR056042. L.P. and L.K. are supported by a senior investigator grant from the European Research Council. S.R. is supported by NIH grants R01AR063759-01A1 and K08-KAR055688A. P.M.V. is a National Health and Medical Research Council Senior Principal Research Fellow. M.A.B. is funded by the National Health and Medical Research Foundation Senior Principal Research Fellowship, and a Queensland State Government Premier’s Fellowship. H.X. is funded by the China Ministry of Science and Technology (973 program grant 2011CB946100), the National Natural Science Foundation of China (grants 30972339, 81020108029 and 81273283), and the Science and Technology Commission of Shanghai Municipality (grants 08XD1400400, 11410701600 and 10JC1418400). K.A.S. is supported by a Canada Research Chair, The Sherman Family Chair in Genomics Medicine, Canadian Institutes for Health Research grant 79321 and Ontario Research Fund grant 05-075. S.M. is supported by Health and Labour Sciences Research Grants. The BioBank Japan Project is supported by the Ministry of Education, Culture, Sports, Science and Technology of the Japanese government. This study is supported by the BE THE CURE (BTCure) project. We thank K. Akari, K. Tokunaga and N. Nishida for supporting the study.
Author information
Authors and Affiliations
Consortia
Contributions
Y.O. carried out the primary data analyses. D.W. managed drug target gene data. G.T. conducted histone mark analysis. T.R., H.-J.W., T.E., A.M., B.E.S., P.L.D. and L.F. conducted eQTL analysis. C.T., K.I., Y.K., K.O., A.S., S.Y., G.X., E.K. and K.A.S. conducted the de novo replication study. R.R.G., A.M., W.O., T.B., T.W.B., L.J., J. Yin, L.Y., D.-F.S., J. Yang, P.M.V., M.A.B. and H.X. conducted the in silico replication study. E.A.S., D.D., J.C., T.K., R.Y. and A.T. managed GWAS data. All other authors, as well as the members of the RACI and GARNET consortia, contributed to additional analyses and genotype and clinical data enrolments. Y.O. and R.M.P. designed the study and wrote the manuscript, with contributions from all authors on the final version of the manuscript.
Corresponding authors
Ethics declarations
Competing interests
R.R.G., A.M., W.O., T.B. and T.W.B. are employees of Genentech. P.P.T. is an employee of GlaxoSmithKline. R.M.P. is currently employed by Merck & Company. The other authors declare no competing financial interests.
Additional information
Summary statistics from the GWAS meta-analysis, source codes, and data sources used in this study are available at http://plaza.umin.ac.jp/~yokada/datasource/software.htm.
Lists of participants and their affiliations appear in the Supplementary Information.
Lists of participants and their affiliations appear in the Supplementary Information.
Extended data figures and tables
Extended Data Figure 1 An overview of the study design.
a, We conducted a three-stage trans-ethnic meta-analysis in total of 29,880 RA cases and 73,758 controls of European (EUR) and Asian (ASN) ancestry. The stage 1 GWAS meta-analysis included 19,234 RA cases and 61,565 controls from 22 studies, which was followed by the stage 2 in silico replication study (3,708 RA cases and 5,535 controls) and stage 3 de novo replication study (6,938 RA cases and 6,658 controls). In the combined study of stages 1–3, we identified 42 novel RA risk loci, which increased the total number of RA risk loci to 101. b, Using the 100 RA risk loci (outside of the MHC region), we conducted trans-ethnic and functional annotation of the RA risk SNPs. We constructed an in silico bioinformatics pipeline to prioritize biological candidate genes. We adopted eight criteria to score each of 377 genes in the RA risk loci: (1) RA risk missense variant; (2) cis-eQTL; (3) PubMed text mining; (4) PPI; (5) PID; (6) haematological cancer somatic mutation; (7) knockout mouse phenotype; and (8) molecular pathway. Our study also demonstrated that these biological candidate genes in RA risk loci are significantly enriched in overlap with target genes for approved RA drugs.
Extended Data Figure 2 Quantile–quantile plots and Manhattan plots of P values in the GWAS meta-analysis.
a, Quantile–quantile plots of P values in the stage 1 GWAS meta-analysis for trans-ethnic, European and Asian ancestries. The x-axis indicates the expected −log10 (P values). The y-axis indicates the observed −log10 (P values) after the application of double GC correction. The SNPs for which observed P values were less than 1.0 × 10−20 are indicated at the upper limit of each plot. Black, blue and red dots represent the association results of all SNPs, SNPs outside of the MHC region and PTPN22 locus, and SNPs outside of the known RA risk loci, respectively. Double GC correction was applied based on the inflation factor, λGC, which was estimated from the SNPs outside of the known RA loci and indicated in each plot. b, Manhattan plots of P values in the stage 1 GWAS meta-analysis for trans-ethnic, European and Asian ancestries. The y-axis indicates the −log10 (P values) of genome-wide SNPs in each GWAS meta-analysis. The horizontal grey line represents the genome-wide significance threshold of P = 5.0 × 10−8. The SNPs for which P values were less than 1.0 × 10−20 are indicated at the upper limit of each plot.
Extended Data Figure 3 Trans-ethnic and functional annotation of RA risk SNPs.
a, b, Comparisons of RAF and OR values between individuals of European (EUR) and Asian (ASN) ancestry from the stage 1 GWAS meta-analysis. ORs were defined based on minor alleles in Europeans. SNPs with FST > 0.10 or SNPs in which the 95% CI of the OR did not overlap between Europeans and Asians are coloured. OR of the SNP in the HLA-DRB1 locus (≥1.5) is plotted at the upper limits of the x- and y-axes. Five loci demonstrated population-specific associations (P < 5.0 × 10−8 in one population but P > 0.05 in the other population without overlap of the 95% CI of the OR) are highlighted by red labels (rs227163 at TNFRSF9, rs624988 at CD2, rs726288 at SFTPD, rs10790268 at CXCR5 and rs73194058 at IFNGR2). c, Cumulative curve of explained heritability in each population. d, Enrichment analysis for overlap of RA risk SNPs with H3K4me3 peaks in cell types. The most significant cell type is Treg primary cells. e, Number of SNPs in the process of trans-ethnic and functional fine mapping. For 31 loci in which the risk SNPs yielded P < 1.0 × 10−3 in both populations (stage 1 GWAS), the number of candidate causal variants was reduced by 40–70% when confined by SNPs in linkage disequilibrium with the RA risk SNPs (r2 > 0.80) in both populations (on average, from 21.9 or 37.3 SNPs in linkage disequiliberium in Europeans or Asians, to 15.0 SNPs in linkage disequilibrium in both populations). Further, for 10 loci in which candidate causal variants significantly overlapped with H3K4me3 peaks in Treg cells (P < 0.05), the average number of SNPs was further reduced by half again, from 10.4 to 5.9. f, Fine mapping in the CTLA4 locus, where the functional non-coding variant of CT60 (rs3087243)28 showed the most significant association with RA. The top three panels indicate regional SNP associations of the locus in the stage 1 GWAS meta-analysis for trans-ethnic, European and Asian ancestries, respectively. The bottom panel indicates the change in the number of the candidate causal variants in each process of fine mapping. Trans-ethnic fine mapping of candidate causal variants decreased the number of candidate variants from 44 (linkage disequilibrium in Asians) and 27 (linkage disequilibrium in Europeans) to 21 (linkage disequilibrium in both populations). As these SNPs were significantly enriched in overlap with H3K4me3 peaks in Treg cells compared with the surrounding SNPs (P = 0.037), we confined the candidate variants into nine by additionally selecting the SNPs included in H3K4me3 peaks. CT60 was included in these finally selected nine SNPs, and also located at the vicinity of a H3K4me3 peak summit (indicated by a red arrow).
Extended Data Figure 4 Pleiotropy of RA risk SNPs.
a, Definition of region-based and allele-based pleiotropy. For each of the RA risk SNPs and SNPs registered in the NHGRI GWAS catalogue (outside of the MHC region), we defined the region on the basis of ±25 kb of the SNP or the neighbouring SNP positions in moderate linkage disequilibrium with it in Europeans or Asians (r2 > 0.50). We defined ‘region-based pleiotropy’ as two phenotype-associated SNPs sharing part of their genetic regions or any UCSC hg19 reference gene(s) partly overlapping with each of the regions. We defined ‘allele-based pleiotropy’ as two phenotype-associated SNPs in linkage disequilibrium in Europeans or Asians (r2 > 0.80). b, Region-based pleiotropy of the RA risk loci. We found two-thirds of RA risk loci (n = 66) demonstrated region-based pleiotropy with other human phenotypes. Phenotypes which showed region-based pleiotropy with RA risk loci are indicated (P < 0.05). c, Allele-based pleiotropy of the RA risk loci. Allele-based pleiotropy with discordant directional effects to RA risk SNPs are indicated in grey. d, Relative proportions of pleiotropic effects (that is, regions and alleles that influence multiple phenotypes) between RA risk loci and 311 phenotypes from the NHGRI GWAS catalogue. Representative examples of disease and biomarker phenotypes are shown. One-quarter of the observed region-based pleiotropic associations (26% = 54/207) were also annotated as having allele-based pleiotropy, although their proportions and directional effects varied among phenotypes. e, Allele-based pleiotropy of IL6R 358Asp (rs2228145 (A))5 on multiple disease phenotypes, including increased risk of RA, ankylosing spondylitis and coronary heart disease (asterisks indicate associations obtained from the literature29,30) and protection from asthma, as well as levels of biomarkers (increased C-reactive protein (CRP) and fibrinogen but decreased soluble interleukin-6 receptor (sIL6R)).
Extended Data Figure 5 Overlap of RA risk SNPs with biological resources.
a, Missense variants in linkage disequilibrium (r2 > 0.80 in Europeans or Asians) with RA risk SNPs. When multiple missense variants are in linkage disequilibrium with the RA risk SNP, the highest r2 value is indicated. b, Functional annotation of the SNPs in 100 non-MHC RA risk loci, including the relative proportion of heritability explained by SNP annotations. Although 44% of all RA risk SNPs had cis-eQTL, 9 of them overlapped with missense or synonymous variants but 35 of them did not overlap as indicated by asterisks. A list of cis-eQTL SNPs and genes can be found in Extended Data Table 2. c, Overlap of RA risk genes with human PID and defined categories. d, Overlap of RA risk genes with cancer somatic mutation genes. In addition to the categories of all cancers, haematological cancers and non-haematological cancers, cancer types that showed overlap with ≥2 of RA risk genes are indicated. e, Overlap of RA risk genes with knockout mouse phenotypes. Knockout mouse phenotypes that satisfied significant enrichment with RA risk genes are indicated in bold (P < 0.05/30 = 0.0017). f, Molecular pathway analysis of RA GWAS results. Molecular pathways that showed significant enrichment in either the current stage 1 trans-ethnic GWAS meta-analysis or the previous GWAS meta-analysis of RA2 are indicated in bold (FDR q < 0.05).
Extended Data Figure 6 Prioritization of biological candidate genes from RA risk loci.
a, Prioritization criteria of biological candidate genes from RA risk loci. b, Histogram distribution of gene scores. The 98 genes with score ≥2 (orange) were defined as ‘biological RA risk genes’. c, Correlations of biological candidate gene prioritization criteria. d, Change in the overlapping proportions of genes with H3K4me3 peaks by cell type according to score increases. When RA risk SNP of the locus (or SNP in linkage disequilibrium) overlapped with H3K4me3 peaks, genes in the locus were defined as overlapping.
Extended Data Figure 7 Overlap of all genes in the RA risk loci with drug target genes.
a, Approved RA drugs and target genes. DMARDs, disease-modifying antirheumatic drugs. b, Overlap analysis stratified by immune-related and non-immune-related drug target genes. We made a list of 583 immune-related genes based on Gene Ontology (GO) pathways named ‘immune-’ or ‘immuno-’ and found that the majority of drug target genes (791/871 = 91%) were not immune-related. c, Overlap of all 377 genes included in 100 RA risk loci (outside of the MHC region) plus 3,776 genes in direct PPI with them and drug target genes. We found overlap of 19 genes from the 27 drug target genes of approved RA drugs (2.3-fold enrichment, P < 1.0 × 10−5). All 871 drug target genes (regardless of disease indication) overlap with 329 genes from the PPI network, which is 1.3-fold more enrichment than expected by chance alone (P < 1.0 × 10−5), but less than 1.7-fold enrichment compared with RA drugs (P = 0.0059). We note that this enrichment of drug–gene pairs was less apparent compared with that obtained from the expanded PPI network generated from 98 biological candidate genes (Fig. 3b).
Extended Data Figure 8 Connection between RA risk genes and approved RA drugs.
Full lists of the connections between RA risk SNPs (blue boxes), biological candidate genes from each risk locus (purple boxes), genes from the expanded PPI network (green boxes) and approved RA drugs (orange boxes). Black lines indicate connections. Only IL6R is a direct connection between an SNP–biological gene–drug (tocilizumab)19,20; all other SNP–drug connections are through the PPI network.
Supplementary information
Supplementary Information
This file contains Supplementary Tables 1-6 and a Supplementary Note. (PDF 449 kb)
Supplementary Data
This file contains the source data file for Supplementary Table 2. (XLSX 10 kb)
Supplementary Data
This file contains the source data file for Supplementary Table 3. (XLSX 24 kb)
Supplementary Data
This file contains the source data file for Supplementary Table 4. (XLSX 2583 kb)
Supplementary Data
This file contains the source data file for Supplementary Table 5. (XLSX 51 kb)
Supplementary Data
This file contains the source data file for Supplementary Table 6. (XLSX 17 kb)
Rights and permissions
About this article
Cite this article
Okada, Y., Wu, D., Trynka, G. et al. Genetics of rheumatoid arthritis contributes to biology and drug discovery. Nature 506, 376–381 (2014). https://doi.org/10.1038/nature12873
Received:
Accepted:
Published:
Issue Date:
DOI: https://doi.org/10.1038/nature12873